Lung cancer is the leading cause of cancer-related deaths, with up to 20 percent of cases occurring in people who have never smoked. Researchers have been working on ways to improve lung cancer risk prediction in never-smokers, and a recent study has shown promising results using deep learning technology.
The study, conducted by Boston University School of Medicine, aimed to determine whether a deep learning model could effectively identify never-smokers at high risk for lung cancer based on their chest X-rays from electronic medical records. Deep learning, a form of advanced artificial intelligence, can be trained to analyze X-ray images and detect patterns associated with disease.
Lead author Anika S. Walia, a medical student at Boston University, explained that their approach only required a single chest X-ray image, making it a simple and widely available test in medicine. The “CXR-Lung-Risk” model was developed using over 147,000 chest X-rays of asymptomatic smokers and never-smokers to predict lung-related mortality risk based on a single chest X-ray image.
The model was then validated in a separate group of never-smokers who had routine outpatient chest X-rays. The results showed that the deep learning model could accurately identify individuals at high risk for lung cancer. In fact, the high-risk group identified by the model had a significantly greater risk of developing lung cancer compared to the low-risk group.
Michael T. Lu, the director of artificial intelligence and co-director of Cardiovascular Imaging Research Center (CIRC) at Massachusetts General Hospital, emphasized the potential of this AI tool for opportunistic screening of never-smokers at high risk of lung cancer, using existing chest X-rays in electronic medical records.
The declining rates of cigarette smoking make early detection of lung cancer in non-smokers increasingly important, and this innovative approach could play a crucial role in identifying at-risk individuals for timely intervention.
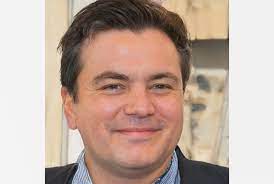
I have over 10 years of experience in the cryptocurrency industry and I have been on the list of the top authors on LinkedIn for the past 5 years. I have a wealth of knowledge to share with my readers, and my goal is to help them navigate the ever-changing world of cryptocurrencies.